Explained: Marketing Mix Modelling
TLDR: Marketing Mix Modelling (MMM) is a statistical analysis technique that lets marketers measure the impact of their marketing and advertising campaigns to determine how various elements contribute to their objection. The insights help optimise ad spends and allow marketing teams to refine their efforts based on various factors that contribute to conversion or engagement In […]
Topics
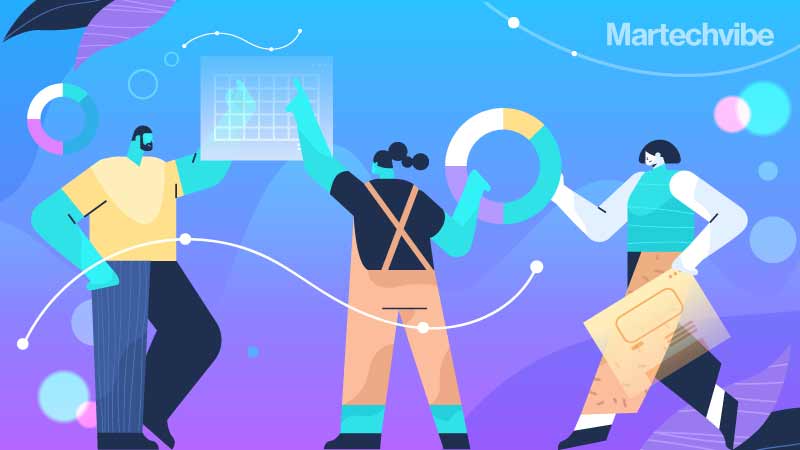
TLDR: Marketing Mix Modelling (MMM) is a statistical analysis technique that lets marketers measure the impact of their marketing and advertising campaigns to determine how various elements contribute to their objection. The insights help optimise ad spends and allow marketing teams to refine their efforts based on various factors that contribute to conversion or engagement
In 2018, when Hershey’s marketing team was tasked with driving sales for Reese’s Peanut Butter Cups, they turned to media partners and their media agency to supply data directly to their marketing mix modelling brand’s measurement partner. The insights from the report pointed to how six-second bumper ads were the most effective in driving sales for this particular product line. With this information, The Company’s portfolio of brands increased 40 per cent year over year from 2017 to 2018.
Marketing Mix Modelling (MMM) is a statistical analysis technique that lets marketers measure the impact of their marketing and advertising campaigns to determine how various elements contribute to their objection. The insights help optimise ad spends and allow marketing teams to refine their efforts based on various factors that contribute to conversion or engagement depending on the goal.
It’s interesting to note that the term marketing mix was developed by Neil Borden, a professor of advertising at Harvard University, in 1949. Traditionally, marketing has looked at the marketing mix as a recipe of ingredients to deliver winning campaigns. These were the early days of advertising when author and professor E. Jerome McCarthy introduced the concept of the four P’s of marketing – price, promotion, product and place as variables that heavily influenced a brand’s marketing strategy. Advertisers then studied how each factor played a significant role and estimated the success of the campaign.
Also Read: Can Privacy And Data-Driven Marketing Co-Exist?
Over the past decade, large consumer packaged goods companies like P&G, AT&T, Kraft, Coca-Cola and Pepsi have made MMM an integral part of their marketing planning. These companies have been leading the charge in studying market dynamics and how they affect product launches across SKUs and new geographic expansions. Vendors that offer marketing mix modelling solutions include Facebook, Nielsen, Mass Analytics, Dunn Solutions, MarketShare, Marketing Management Analytics, Analytic Partners, Marketing Evolution and ThinkVine, Ninah, Hudson River Group, IRI and many more. Algorithms have since replaced the need for manual collation of data and analysis.
In the digital age, every company has a mechanism in place to collect and analyse data. Data drives such important decisions such as allocating funds and offers a clearer view to measure success using historical sales figures and attribution metrics. Today, marketers can ascertain the effectiveness of each marketing input in terms of Return on Investment.
MMM uses Regression techniques and analysis to determine the relationship between base and incremental variables in producing a particular outcome.
In the MMM equation, Base Sales are defined as the default sales that the brand would achieve if they do not use any advertisements. It is attributed to sales due to brand equity built over the years. Base Sales are usually fixed unless acted upon by economic or environmental factors.
Base Drivers are elements like price, distribution, seasonality and macroeconomic factors that influence sales. Price is a factor that affects decision-making based on the perceived value and competitor landscape, distribution refers to the number of stores, location and inventory available, seasonality refers to critical times of the year when demand is at its peak, and macro-economic factors caters to GDP, unemployment rate, purchase power, growth rate, inflation and consumer sentiment and such. Historical data informs marketing teams about the influence of such factors, which remain mostly unchanged. The pandemic changed these baselines for most products.
Also Read: Marketing Mix Modelling Re-Discovered!
Next, models look at Incremental Sales or sales generated due to marketing activities like TV and print advertisements and digital spending. Total incremental sales are split into sales from each input to calculate contribution to total sales.
Incremental Drivers include media promotions, product launches, behavioural and social media metrics. Over the years, these parameters have increasingly gained more importance in their influence on consumer buying behaviour. Each marketing effort feeds the model, which means the measurement of each channel needs to be trustworthy. For example, social listening tools measure sentiment towards the brand on social media. This informs the model how social media efforts can either correct a negative sentiment or play up positive ones. It is also a way to identify under-performing assets that may be using up funds.
Why is this important? According to ad-stock theory, advertising is not immediate and has diminishing returns. This means that its influential power decreases over time even if more funds are allocated to it. Time regression analysis helps marketers find that sweet spot and establish a potential timeline to optimise media buying. Similarly, the carry-over effect refers to the impact of past advertisements on present sales. It is calculated as Gross Rating Point or GRP, which measures the impact of advertisements. MMM helps account for how these factors influence current efforts. Depending on whether a brand follows a branded house or house of brands approach, it can also calculate the cannibalisation effect, which refers to the negative impact on a product from a brand because of the performance of other products from the same brand. The reverse of this is called the halo effect, where products face positive sentiment due to good experiences with other products in the line. In MMM, base variables or incremental variables of other products of the same brand are tested to understand the halo or cannibalising impact on the business outcome of the product under consideration.
One of the key objectives of MMM is to find the outliers and explain what caused these spikes. With this knowledge, brands can make more informed decisions about how to ride on the goodwill of previous sister product lines, tackle the competition and make optimal use of budgets without wastage.
Apart from validating current marketing efforts, MMM can play a significant role in testing experimental plans, which makes it a forecasting tool as well.